Cedar Creek Wind Farm–Cary Leppert, Shutterstock.com
How the Wind Blows
NEXUS researchers investigate improved wind forecasting to harness its clean energy potential
By Jane Palmer
January, 2018
From a gentle breeze to a full out gale, wind represents a clean and sustainable form of energy but harnessing the full potential of wind power can be challenging. “Basically, the wind is controlled by nature, It means that you cannot simply control the generation output of a wind farm,” says NEXUS researcher Dr. Lei Yang, a professor of computer science and engineering at the University of Nevada, Reno.
Wind is a highly variable and intermittent source of energy, which means that integrating it into the power system can prove tricky. Power companies engage in a delicate balancing act, day in and day out, to balance energy demand and energy supply. The grid currently operates on a 60 hertz frequency – if not enough energy is available to meet this demand the result can be blackouts or brownouts. Therefore, when integrating wind energy into the power system, power companies will rely heavily on backups to manage against the sudden changes in wind energy.
To deploy this juggling act efficiently requires accurate forecasts of when the wind levels will rise and fall. But, at present, current wind forecasts are highly inaccurate. Yang’s goal is use a combination of big data analytics and statistical models to provide more accurate and therefore valuable estimates of wind power. “We cannot control wind, but we can forecast wind,” Yang says. “So if we can do a good job in the forecasting then pretty much we can achieve our mission of pure, clean energy in the end.”
Big Data Analytics and Statistical Models–L. Yang
Predicting a Chain of Events
In a single hour, air speed can go from stationary to blustery and back to calm. An effective wind forecast will predict each step in this chain of events. To do so accurately Yang used a model called a “Markov Chain” that is especially good at estimating how a system changes over time.
Initially, Yang needed to teach the model what has happened historically. To do this he “trained” the model on a massive data set from a large wind farm. Sensors on this wind farm record the wind speed and direction and instruments also record the output of each of the 300 wind turbines every 10 minutes. “Basically, we take all the data, and build this chain,” Yang says.
Given a particular wind speed, the model estimates the probability of the wind being a certain speed in the next instance. As such the prediction method is based on the historical correlations between one wind speed and the next. “Based on the experiments using real data from a wind farm in the US, we show that our approach can significantly improve the prediction accuracy,” Yang says.
Wind Farm Spatial Dynamics–L. Yang
Ramping Up the Accuracy
Another challenge, however, faces scientists in trying to forecast what the wind speed will be from one moment to another: wind ramps. Wind ramps are extremely sudden, drops or increases in wind speed, and a sudden drop in wind speed could result in power outages if it takes power system operators by surprise. In some large wind energy plants wind ramps can occur approximately every two days, so it is important to forecast when they might occur for efficient grid operations, Yang says.
To estimate when wind ramps may occur Yang augmented the Markov Chain model with an additional “Support Vector Machine” (SVM) module that is also trained on historical data. This module estimates the probability of a wind ramp event at any instant in time. If it detects a high probability of a wind ramp event it will estimate the change in wind power. If it detects a low probability of a wind ramp event, the Markov Chain model will estimate the wind power. Yang found this combined approach produced more accurate forecasts of wind generation, especially for the prediction of wind ramps.
Although their forecasting method has shown a demonstrable improvement over the predictions of other methods, there is still much room for improvement, Yang says. Patterns of wind change on a seasonal and daily basis and to take this variation into account the scientists use multiple models for different seasons and different times of day. “So we will use a model in the morning, and another model in the afternoon, for example,” Yang says. “And use a model in the month of January and another model in February.”
This approach assumes that the wind speeds and fluctuations are the same from one year to the next for given months of the year. But some years, March,for example,may always be a windy month, but could be more, or less windy than normal. “We kind of divide the time into different periods and use a static model for each period,” Yang says. “But the problem is that the way the wind process behaves is not always static.”
Consequently the team is now working on making their approach more dynamic by developing a detection algorithm. This algorithm first tests to see if the wind speed is “different from normal” and, if it is, a new model is used to forecast the wind. In addition, the team is also planning on using more advanced techniques and machine learning to incorporate the extremely random nature of the wind and to improve on the accuracy of their forecasts.
SVM Enhanced Markov Forecast, Distribution Forecast, January 30, 2010–L. Yang
Calculating the Bottom Line
Ultimately the goal in integrating renewable energy into the power system is to meet the needed power demand, using the maximum amount of renewable energy at the lowest possible cost.
“So the idea is trying to optimize the system output in an economic way,” Yang says. Within each power system, there are many generators and the objective is to operate them in such a way that the cost of the system is minimized, Yang says. To attempt to find this optimal operating point Yang and his team used a stochastic Economic Dispatch model.
This model uses the forecast of the wind at projected future intervals and the probability of this value occurring to determine how best to maximize the productivity of the system. Simulations using this model have been shown to improve the efficiency of the system. “It can improve the system cost by several percentage points,” Yang says. “Although the percentage itself may not look high, the dollar savings are huge because it is a billion dollar market.”
Renewable Portfolio Standard (RPS) goals of renewable generation integration–L. Yang
–NSF’s Big Ideas
_______________________________________
Harnessing the Data Revolution for Renewable Energy
_______________________________________
In 2016, the National Science Foundation (NSF) unveiled a set of “Big Ideas” – ten futuristic and long term research and process ideas at the frontiers of science and engineering. One of these ideas is to develop a cohesive, national-scale approach to research data infrastructure, which includes harnessing the power of big data. This initiative will enable data-driven discovery through visualization, better data mining, and machine learning, and train the next generation of data scientists. “We are proud that Dr. Yang, who was hired by the NEXUS project, is contributing to NSF’s Initiative by using big data to address challenges in the efficient integration of renewable energy into the power system infrastructure” says Dr. Gayle Dana, NEXUS Project Director.
_______________________________________
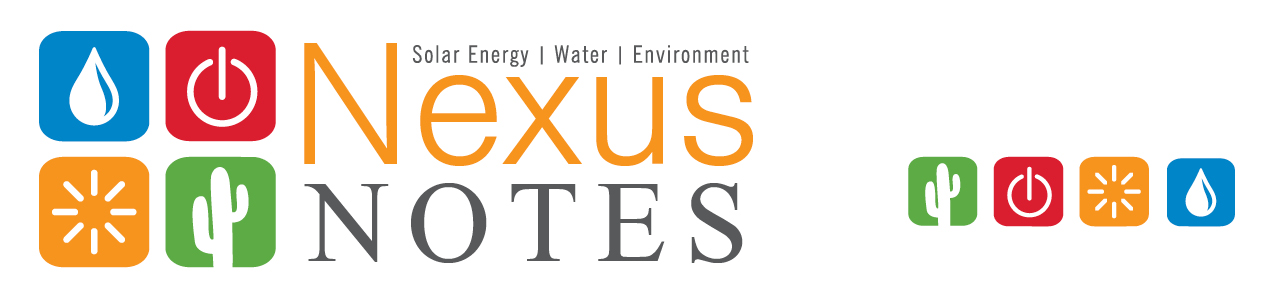
NEXUS Notes is a monthly publication of the Solar Nexus Project, which is a five-year research project funded by the National Science Foundation’s Established Program to Stimulate Competitive Research “EPSCoR” (Cooperative Agreement #IIA-1301726) focusing on the nexus of (or linkage between) solar energy generation and Nevada’s limited water resources and fragile environment.
Any opinions, findings, and conclusions or recommendations expressed in this material are those of the author(s) and do not necessarily reflect the views of the National Science Foundation.
_______________________________________
If you would like to know more about the NEXUS project,
please contact, Dr. Gayle Dana
Gayle.Dana@dri.edu
530-414-3170
_______________________________________